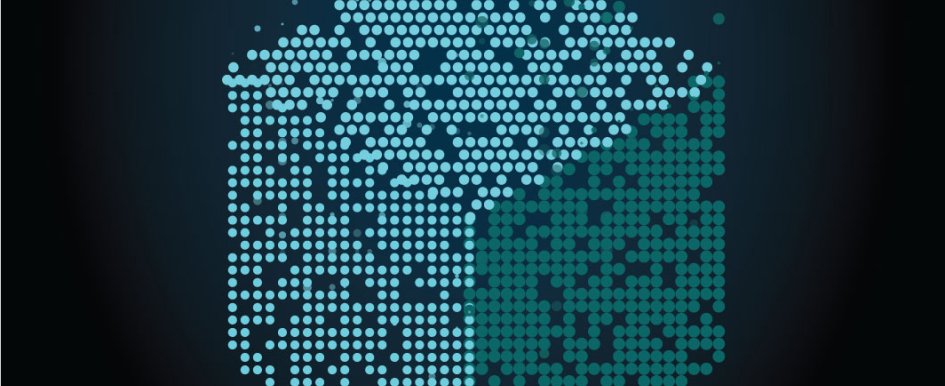
Preconstruction planning is one of the most challenging aspects of the construction life cycle, and it has been for quite some time. But that’s all changing, in part due to data science and the predictive-cost model.
Conventionally, business owners, facility managers and construction professionals relied on historic prices and localization factors to provide reasonable, accurate costs to build. While these costs and factors were helpful in creating budgets, stakeholders grew dissatisfied with the lack of accuracy in the approach. Between market volatility, unpredictable commodities prices and a shrinking labor pool, historical data is simply unreliable.
Access to predictive data that accounts for real market conditions is critical for budget management, from design through construction. Data science now empowers business owners to supplement historical data with reliable cost projections.
Predictive Cost vs. Traditional Forecast
Predictive-cost data addresses the shortcomings of traditional forecast data. It was created and is maintained using a hybrid methodology that combines classical econometric techniques with contemporary data-mining methodology. Although it is based on econometric principles and modeling techniques, predictive-cost data differs from traditional econometric forecasts in two ways.
First, traditional econometric forecasts are based on macroeconomic theory, even when analyses of historical values of those macroeconomic indicators demonstrate them to be statistically insignificant predictors. Predictive-cost models disregard theory and are based exclusively on data-driven, empirical evidence instead. This empirical evidence is the result of extensive exploratory data analyses and pattern-seeking visualizations of historical cost data with economic and market indicators prior to model development.
This approach, an update to the centuries-old, theory-driven process, has been extensively researched and validated by Dr. Edward Leamer, professor of global economics and management at The University of California, Los Angeles (UCLA). Only economic indicators that have proven themselves in exploratory analyses become candidates for model development, testing, validation and resulting predictive-cost estimates.
Second, predictive-cost data uses mining techniques and principles to improve traditional econometric modeling practices. Since the 1990s, this family of processes and analyses has evolved from a mix of classical statistical principles to more contemporary computer science and machine-learning methods.
Data-mining methodology is specifically designed to analyze observational data instead of experimental data, as in classical statistical and econometric techniques. It is a robust methodology that takes advantage of recent increases in computing power, data-visualization techniques and updated statistical procedures to find patterns and determine structural drivers of construction material and labor costs.
Measures of these drivers and their relationships to each other and to construction costs (along with their associated lead or lag times) are then represented in a statistical algorithm that predicts future values for defined materials and locations.
Budgeting with Predictive-Cost Data
One of the big challenges for design and construction teams is managing the budget. Predictive data does more than give an estimate of the total cost and scale them over time; it allows you to optimize the entire build schedule and has been used to predict the cost of construction up to 3 years before the project breaks ground.
Conceptual square-foot models are normally within 20 percent of actual costs, but applying a true predictive multivariate database—including individual material, labor and equipment data—can improve the accuracy of a conceptual estimate, allowing owners, architects, engineers and other construction professionals the ability to plan projects more confidently.
Ultimately, the core value of using predictive-cost data at the material, labor and equipment levels is the unprecedented ability to understand future costs for better planning. Predictive-cost data will not solve every preconstruction planning difficulty, but it is a leap forward in terms of estimate accuracy and preplanning efficiency.